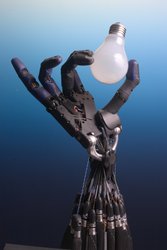
Leslie Pack Kaelbling
The American roboticist Leslie Pack Kaelbling is a panasonic professor of computer science and engineering at the Massachusetts Technology Institute. She is widely known for adapting Markov’s partially observable decision-making process for operational research for artificial intelligence and robotic application. In 1997, Kaelbling received the IJCAI Computers and Thought Award for enhanced learning in embedded control systems and the development of robot navigation programming tools. In 2000, she was elected Fellow of the Artificial Intelligence Association.
Kaelbling got an A. B. B. In 1983 in Philosophy and Ph.D. In 1990, both at Stanford University in Computer Science. During this time she also became a member of the Center for Language and Information Studies. Before joining Brown University, she then worked at SRI International and the associated robotics spin-off Teleos Research. In 1999, she left Brown to join the professorship at MIT. Her research focuses on policymaking, machine learning and sensing with robotics applications.
She and two-thirds of the Kluwer-owned journal Machine Learning, both in Spring 2000, resigned in protest at their pay-to-access archives, while the financial compensation for authors was simultaneously limited. Kaelbling co-founded and served as the Journal of Machine Learning Research’s first editor-in-chief, an open access journal reviewed by peers, covering the same topics. It permits researchers to freely publish and retain copyright in their online archives. In response to the mass resignation, Kluwer amended its publication policy to enable authors to archive their papers online after an online review by peers. Kaelbling replied that this policy was reasonable and would have made the creation of an alternative journal unnecessary, but it was not until the threat of resignation and the founding of the JMLR that the publication policy changed, that the Editorial Board made it clear that they wanted such a policy.