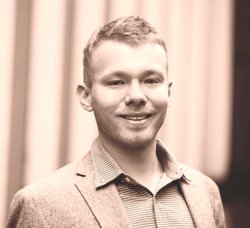
Wojciech Zaremba
Wojciech Zaremba is the OpenAI co-founder, leading the robotics team. His team is developing general purpose robots through new approaches to transferring complicated learning and teaching robots. OpenAI’s mission is to create safe artificial intelligence and ensure its advantages are distributed as evenly as possible.
Zaremba was born in Poland, in Kluczbork. He won local competitions and awards in mathematics, IT, chemistry and physics at a young age. Zaremba represented Poland at the International Olympiad in 2007 and won an Silver Medal in Vietnam. Zaremba studied mathematics and computer-science at the University of Warsaw and at the École Polytechnique and graduated in mathematics in 2013. He then started his doctorate in profound education at New York University under Yann LeCun and Rob Fergus. Zaremba graduated in 2016, and was awarded his PhD.
He spent time at NVIDIA during the pre-deep education period during his bachelor’s studies. NVIDIA chips were developed in the following years
A pillar of artificial intelligence. His PhD was divided between a year spent in Google brain and another year spent in Facebook Artificial Intelligence Research.
During his stay at Google, he co-authored work on opponents of neural networks. This result created the field of neural network adversarial attacks. His Ph.D. focuses on matching neural network capabilities to algorithmic computer power. The problem of training neural networks to represent a programmable computer algorithm was turned into a separate field.
In 2015, Zaremba joined OpenAI, a non-profit research firm for artificial intelligence. The project’s objective is to create safe artificial intelligence. The headquarters in San Francisco has a budget of one billion dollars. Zaremba works as Robotics Research Manager at OpenAl. Zaremba sits on the consultancy board of Growbots, a start-up firm in Silicon Valley that aims to automate machine learning and artificial intelligence sales processes.
Zaremba has published over 40 publications devoted to machine learning and artificial intelligence with several thousand quotes.
30 the Polish magazine “Forbes” 2017, the most influential before the 30s.
48th International Mathematical Olympiad, Vietnam Silver Medal
Polish Children’s Fund Scholar from 2000 to 2007
Aleksander Kwaśniewski President Skilful Children’s Scholarship