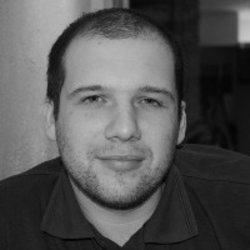
Razvan Pascanu
Research Scientist at Google DeepMind, Phd Student in Machine Learning at Université de Montréal, Developer at Université de Montréal
Research Scientist at Google DeepMind, Phd Student in Machine Learning at Université de Montréal, Developer at Université de Montréal
Use your Google Account to sign in to DeepAI