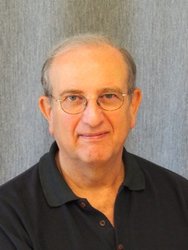
John K. Tsotsos
Tsotsos received an Hons. B.A.Sc. in Engineering Science in 1974, an M.Sc. in Computer Science in 1976 and a PhD. in Computer Science in 1980, all from the University of Toronto.
He then joined the University of Toronto on faculty in both Departments of Computer Science and of Medicine. He founded the Computer Vision Group at the University of Toronto in 1980, which he led until 1999.
He moved to York University in 2000 where he is now Distinguished Research Professor of Vision Science. He was Director of the Centre for Vision Research at York University from 2000-2006. Among his honors are: Canadian Heart Foundation Scholar at University of Toronto 1981-1984; an Honorable Mention Marr Prize at the 1st International Conference in Computer Vision in 1987; Fellow, Artificial Intelligence and Robotics Program at Canadian Institute for Advanced Research 1985-1995; Tier I Canada Research Chair of Computational Vision 2003-2024; Fellow of the Royal Society of Canada; Fellow IEEE; 2006 Canadian Image Processing and Pattern Recognition Society Award for Research Excellence and Service; the 1st President’s Research Excellence Award from York University on the occasion of the University’s 50th anniversary in 2009; the 2011 Geoffrey J. Burton Memorial Lectureship from the United Kingdom's Applied Vision Association for significant contribution to vision science; the 2015 Sir John William Dawson Medal from the Royal Society of Canada for sustained excellence in multidisciplinary research, the first computer scientist to be so honored; and several conference best paper or finalist awards.
His current research focuses on a comprehensive theory of visual attention in humans. A practical outlet for this theory forms a second focus, embodying elements of the theory into the vision systems of mobile robots.