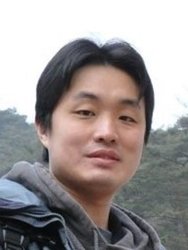
Yu-Wing Tai
Research Director of Youtu Lab at Tencent since 2017, Principal Researcher at SenseTime Group Limited 2015-2016, Adjunct Associate Professor at the Department of Computer Science and Engineering, HKUST, Associate professor at the Korea Advanced Institute of Science and Technology (KAIST) from July 2009 to August 2015, Assistant Professor at KAIST from 2009-2014, full-time student internship in the Microsoft Research Asia (MSRA) 2007-2008, Microsoft Research Asia Fellowship in 2007, and the KAIST 40th Anniversary Academic Award for Excellent Professor in 2011 respectively, PhD degree from the National University of Singapore in 2009.