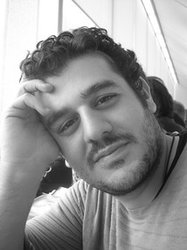
Stefanos Zafeiriou
Reader in Machine Learning and Computer Vision at Imperial College London, Senior Lecturer in Computer Vision/Statistical Machine Learning at Imperial College London from 2015-2017, Lecturer in Computer Vision/Statistical Machine Learning at Imperial College London from 2012-2015, Research Fellow (Junior Research Fellowship) at IImperial College London from 2011-2012, Research Fellow at Imperial College London from 2010-2011, Research Associate at Deparment of Computing, Imperial College London 2010, Research Associate at Department of Electrical and Electronic Engineering, Imperial College London from 2008-2010, Visitor at University of Houston 2009, PhD in Computer Vision and Statistical Machine Learning at Aristotle University of Thessaloniki