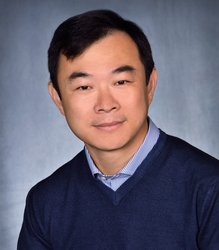
Eric P Xing
Professor of Machine Learning, Language Technology, Computer Science, Cargenie Mellon University
Professor of Machine Learning, Language Technology, Computer Science, Cargenie Mellon University
Use your Google Account to sign in to DeepAI