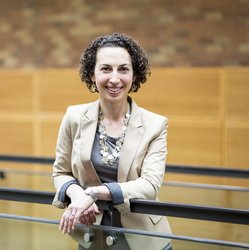
Emily B. Fox
Associate Professor in the Paul G. Allen School of Computer Science & Engineering and Department of Statistics at the University of Washington
Associate Professor in the Paul G. Allen School of Computer Science & Engineering and Department of Statistics at the University of Washington
Use your Google Account to sign in to DeepAI