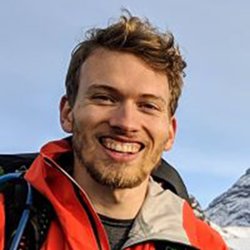
Curtis G. Northcutt
About
I am graduating this spring (2021) with my PhD in EECS at MIT advised by Isaac Chuang, and supported by an NSF Fellowship and a MITx Digital Learning Research Fellowship.
My research focuses on two goals: (1) dataset uncertainty estimation, (2) the synergy of artificial intelligence to augment human intelligence. To this end, I established confident learning, a family of theory and algorithms for characterizing, finding, and learning with label errors in datasets, and cleanlab, the official Python framework for machine learning and deep learning with noisy labels in datasets. For an overview of my published research, please visit Google Scholar.
In addition to my MIT research, I am Founder & CTO at ChipBrain, the principal author of the L7 machine learning blog, and PomDP the PhD rapper.
In my spare time, I help researchers build affordable state-of-the-art deep learning machines and enjoy competitive mountaineering, hiking, and cycling.
Some awards I’ve received include the MIT Morris Joseph Levin Masters Thesis Award, the NSF GRFP Fellowship, the Barry M. Goldwater National Scholarship, and the Vanderbilt Founder’s Medal (Valedictorian). I created the cheating detection system used by MITx and HarvardX online course teams, particularly in MicroMasters courses. At MIT, I TA’d 6.867, a 400-person advanced graduate machine learning course.
Research Manifesto
- Use machine learning to augment human learning, especially for social good.
- Understand and learn in spite of uncertainty and noise in labeled datasets.
While these two ideas appear disparate, they are mutually dependent. Humans often have false notions about the world and encounter misinformation, yet we still learn well in noisy environments. Augmenting human learning with machine learning necessitates a deeper understanding of learning in noisy environments. Across healthcare, agriculture, politics, economics, transportation… our future as a species relies on an increasing synergy between machine learning and human learning: it is paramount that we have the tools to deal with real-world uncertainty, while maintaining the foresight to focus our advances in machine intelligence towards social good.
Industry and Institutional Research
I am fortunate to have had the opportunity to work or intern at:
- Google AI Research in New York, NY (2019)
- Knowledge AI Startup in Boston, MA (2019-)
- Oculus Research / FRL in Redmond, WA (2018-2019)
- Amazon AI Research in Cambridge, MA (2017)
- Facebook AI Research in New York, NY (2016)
- Microsoft Research India in Bangalore (2014)
- MIT Lincoln Laboratory in Lexington, MA (2013)
- Microsoft in Redmond, WA (2012)
- National Science Foundation NSF REU at Notre Dame (2011)
- General Electric Engineering in Louisville, KY (2010)
- NASA Langley Research Center in Hampton, VA (2009)
as well as academic collaborations and visiting research with MIT, Harvard, Vanderbilt, Notre Dame, and the University of Kentucky.