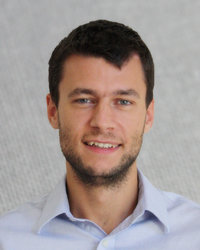
Stefano Ermon
Assistant Professor, Department of Computer Science Fellow, Woods Institute for the Environment at Stanford University
Assistant Professor, Department of Computer Science Fellow, Woods Institute for the Environment at Stanford University
Use your Google Account to sign in to DeepAI