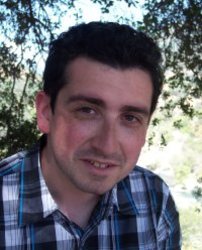
Leonid Sigal
Associate Professor in the Department of Computer Science at the University of British Columbia, Senior Research Scientist at Disney Research Pittsburgh from 2010-2017, Adjunct Faculty member at Carnegie Mellon University, Postdoctoral Researcher at University of Toronto from 2007-2009, Summer Intern at Intel Corporation 2006