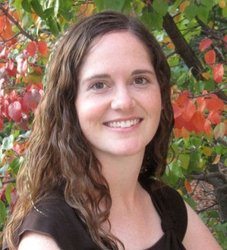
Laura Balzano
Assistant Professor in Electrical Engineering and Computer Science at the University of Michigan
Assistant Professor in Electrical Engineering and Computer Science at the University of Michigan
Use your Google Account to sign in to DeepAI