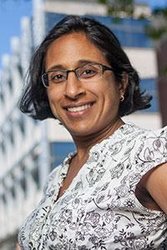
Finale Doshi-Velez
Assistant Professor of Computer Science at Harvard Paulson School of Engineering and Applied Sciences, Harvard University, MSc from the University of Cambridge as a Marshall Scholar, her PhD from MIT, and her postdoc at Harvard Medical School.