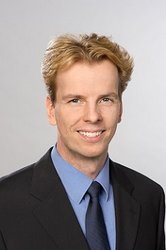
Björn Schuller
Chair of Complex and Intelligent Systems at University of Passau, Full Professor & Head of the Chair of Embedded Intelligence for Health Care and Wellbeing, University of Augsburg, Germany, Reader (Associate Professor) in Machine Learning, Group on Language, Audio & Music, Imperial College London, London/U.K, Chief Executive Officer (CEO) and Co-Founder, audEERING GmbH, Gilching/Germany, Visiting Professor, School of Computer Science and Technology, Harbin Institute of Technology, Harbin/P.R. China.