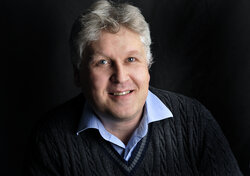
Sepp Hochreiter
Sepp Hochreiter is a German computer scientist. He heads the Institute for Machine Learning and the Linz Institute of Technology AI Lab at the Johannes Kepler University in Linz. He is also a founding director of the Institute of Advanced Research in Artificial Intelligence (IARAI). Previously, he was at Berlin University of Technology, Boulder University in Colorado, and the University of Technology in Munich.
Sepp Hochreiter developed the long short-term memory (LSTM) neural network architecture in his diploma thesis in 1991 followed by the main LSTM publication in 1997. He discovered and analyzed the fundamental deep learning problem of vanishing or exploding gradients. He proposed "Flat Minimum Search" to increase the generalization of neural networks. He suggested modern Hopfield networks with continuous states to equip deep learning architectures with (external) memories.