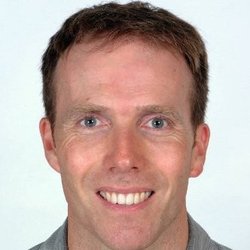
Stephen J. Roberts
Professor of Machine Learning & Director, Oxford-Man Institute of Quantitative Finance at University of Oxford since 1999, CSO & co-founder at Mind Foundry since 2016, Project Scientist of the Machine Learning Research Group, Professorial Fellow of Somerville College, former Director of the EPSRC Centre for Doctoral Training in Autonomous, Intelligent Machines and Systems (AIMS) and Director of the Oxford-Man Institute.